Introduction to Basic Customer Segmentation in Python¶
Problem
Exercise: Expedia Hotel Booking Dataset Customer Segmentation
Read more at kaggle
CEO : My board meeting is coming up next week. I need to present :-
A report of underperforming and overperforming segments
How to tailor the new marketing campaigns for different cities next month?
How to improve the user booking rate?
Imports¶
# import pandas as pd
# import numpy as np
# import scipy as sp
# import seaborn as sns
# import matplotlib as mpl
# import matplotlib.pyplot as plt
from fastai.basics import *
from nlphero.data.external import *
import sklearn as sk
import bqplot as bq
import seaborn as sns
import statsmodels.api as sm
from sklearn.preprocessing import StandardScaler
from sklearn.decomposition import PCA
from sklearn import metrics
from sklearn.cluster import KMeans
from scipy import stats
from ipywidgets import interact, interactive
Read The Data¶
# kaggle competitions download -c expedia-hotel-recommendations
path = untar_data("kaggle_competitions::expedia-hotel-recommendations"); path
Path('/Landmark2/pdo/.nlphero/data/expedia-hotel-recommendations')
path.ls()
(#4) [Path('/Landmark2/pdo/.nlphero/data/expedia-hotel-recommendations/sample_submission.csv'),Path('/Landmark2/pdo/.nlphero/data/expedia-hotel-recommendations/test.csv'),Path('/Landmark2/pdo/.nlphero/data/expedia-hotel-recommendations/train.csv'),Path('/Landmark2/pdo/.nlphero/data/expedia-hotel-recommendations/destinations.csv')]
df_train = pd.read_csv(path/"train.csv", parse_dates=['date_time', 'srch_ci', 'srch_co']); df_train.head()
date_time | site_name | posa_continent | user_location_country | user_location_region | user_location_city | orig_destination_distance | user_id | is_mobile | is_package | ... | srch_children_cnt | srch_rm_cnt | srch_destination_id | srch_destination_type_id | is_booking | cnt | hotel_continent | hotel_country | hotel_market | hotel_cluster | |
---|---|---|---|---|---|---|---|---|---|---|---|---|---|---|---|---|---|---|---|---|---|
0 | 2014-08-11 07:46:59 | 2 | 3 | 66 | 348 | 48862 | 2234.2641 | 12 | 0 | 1 | ... | 0 | 1 | 8250 | 1 | 0 | 3 | 2 | 50 | 628 | 1 |
1 | 2014-08-11 08:22:12 | 2 | 3 | 66 | 348 | 48862 | 2234.2641 | 12 | 0 | 1 | ... | 0 | 1 | 8250 | 1 | 1 | 1 | 2 | 50 | 628 | 1 |
2 | 2014-08-11 08:24:33 | 2 | 3 | 66 | 348 | 48862 | 2234.2641 | 12 | 0 | 0 | ... | 0 | 1 | 8250 | 1 | 0 | 1 | 2 | 50 | 628 | 1 |
3 | 2014-08-09 18:05:16 | 2 | 3 | 66 | 442 | 35390 | 913.1932 | 93 | 0 | 0 | ... | 0 | 1 | 14984 | 1 | 0 | 1 | 2 | 50 | 1457 | 80 |
4 | 2014-08-09 18:08:18 | 2 | 3 | 66 | 442 | 35390 | 913.6259 | 93 | 0 | 0 | ... | 0 | 1 | 14984 | 1 | 0 | 1 | 2 | 50 | 1457 | 21 |
5 rows × 24 columns
Basic Data Exploration¶
df_train.head().T
0 | 1 | 2 | 3 | 4 | |
---|---|---|---|---|---|
date_time | 2014-08-11 07:46:59 | 2014-08-11 08:22:12 | 2014-08-11 08:24:33 | 2014-08-09 18:05:16 | 2014-08-09 18:08:18 |
site_name | 2 | 2 | 2 | 2 | 2 |
posa_continent | 3 | 3 | 3 | 3 | 3 |
user_location_country | 66 | 66 | 66 | 66 | 66 |
user_location_region | 348 | 348 | 348 | 442 | 442 |
user_location_city | 48862 | 48862 | 48862 | 35390 | 35390 |
orig_destination_distance | 2234.26 | 2234.26 | 2234.26 | 913.193 | 913.626 |
user_id | 12 | 12 | 12 | 93 | 93 |
is_mobile | 0 | 0 | 0 | 0 | 0 |
is_package | 1 | 1 | 0 | 0 | 0 |
channel | 9 | 9 | 9 | 3 | 3 |
srch_ci | 2014-08-27 | 2014-08-29 | 2014-08-29 | 2014-11-23 | 2014-11-23 |
srch_co | 2014-08-31 | 2014-09-02 | 2014-09-02 | 2014-11-28 | 2014-11-28 |
srch_adults_cnt | 2 | 2 | 2 | 2 | 2 |
srch_children_cnt | 0 | 0 | 0 | 0 | 0 |
srch_rm_cnt | 1 | 1 | 1 | 1 | 1 |
srch_destination_id | 8250 | 8250 | 8250 | 14984 | 14984 |
srch_destination_type_id | 1 | 1 | 1 | 1 | 1 |
is_booking | 0 | 1 | 0 | 0 | 0 |
cnt | 3 | 1 | 1 | 1 | 1 |
hotel_continent | 2 | 2 | 2 | 2 | 2 |
hotel_country | 50 | 50 | 50 | 50 | 50 |
hotel_market | 628 | 628 | 628 | 1457 | 1457 |
hotel_cluster | 1 | 1 | 1 | 80 | 21 |
df_train.info()
<class 'pandas.core.frame.DataFrame'>
RangeIndex: 37670293 entries, 0 to 37670292
Data columns (total 24 columns):
# Column Dtype
--- ------ -----
0 date_time datetime64[ns]
1 site_name int64
2 posa_continent int64
3 user_location_country int64
4 user_location_region int64
5 user_location_city int64
6 orig_destination_distance float64
7 user_id int64
8 is_mobile int64
9 is_package int64
10 channel int64
11 srch_ci object
12 srch_co object
13 srch_adults_cnt int64
14 srch_children_cnt int64
15 srch_rm_cnt int64
16 srch_destination_id int64
17 srch_destination_type_id int64
18 is_booking int64
19 cnt int64
20 hotel_continent int64
21 hotel_country int64
22 hotel_market int64
23 hotel_cluster int64
dtypes: datetime64[ns](1), float64(1), int64(20), object(2)
memory usage: 6.7+ GB
!ls -la {path}
total 4414434
drwxrwxrwx+ 2 ubuntu ubuntu 0 Oct 25 08:38 .
drwxrwxrwx+ 18 ubuntu ubuntu 0 Oct 25 08:38 ..
-rwxrwxrwx+ 1 ubuntu ubuntu 138159416 Oct 25 08:38 destinations.csv
-rwxrwxrwx+ 1 ubuntu ubuntu 31756066 Oct 25 08:38 sample_submission.csv
-rwxrwxrwx+ 1 ubuntu ubuntu 276554476 Oct 25 08:38 test.csv
-rwxrwxrwx+ 1 ubuntu ubuntu 4070445781 Oct 25 08:40 train.csv
len(df_train)
37670293
# df_train.describe()
Warning
Focus of this exersize is to learn about customer segmentation. We will start with a sample and come back to full dataset after formulating a methodology
Read Sample¶
df_sample = pd.read_csv("https://raw.githubusercontent.com/maoting1223/pycon_sg_2016/master/sample", parse_dates=['date_time', 'srch_ci', 'srch_co'], index_col=0)
df_sample.head().T
24636210 | 19837144 | 13066459 | 4691082 | 4878884 | |
---|---|---|---|---|---|
date_time | 2014-11-03 16:02:28 | 2013-03-13 19:25:01 | 2014-10-13 13:20:25 | 2013-11-05 10:40:34 | 2014-06-10 13:34:56 |
site_name | 24 | 11 | 2 | 11 | 2 |
posa_continent | 2 | 3 | 3 | 3 | 3 |
user_location_country | 77 | 205 | 66 | 205 | 66 |
user_location_region | 871 | 135 | 314 | 411 | 174 |
user_location_city | 36643 | 38749 | 48562 | 52752 | 50644 |
orig_destination_distance | 456.115 | 232.474 | 4468.27 | 171.602 | NaN |
user_id | 792280 | 961995 | 495669 | 106611 | 596177 |
is_mobile | 0 | 0 | 0 | 0 | 0 |
is_package | 1 | 0 | 1 | 0 | 0 |
channel | 1 | 9 | 9 | 0 | 9 |
srch_ci | 2014-12-15 00:00:00 | 2013-03-13 00:00:00 | 2015-04-03 00:00:00 | 2013-11-07 00:00:00 | 2014-08-03 00:00:00 |
srch_co | 2014-12-19 00:00:00 | 2013-03-14 00:00:00 | 2015-04-10 00:00:00 | 2013-11-08 00:00:00 | 2014-08-08 00:00:00 |
srch_adults_cnt | 2 | 2 | 2 | 2 | 2 |
srch_children_cnt | 0 | 0 | 0 | 0 | 1 |
srch_rm_cnt | 1 | 1 | 1 | 1 | 1 |
srch_destination_id | 8286 | 1842 | 8746 | 6210 | 12812 |
srch_destination_type_id | 1 | 3 | 1 | 3 | 5 |
is_booking | 0 | 0 | 0 | 1 | 0 |
cnt | 1 | 1 | 1 | 1 | 1 |
hotel_continent | 0 | 2 | 6 | 2 | 2 |
hotel_country | 63 | 198 | 105 | 198 | 50 |
hotel_market | 1258 | 786 | 29 | 1234 | 368 |
hotel_cluster | 68 | 37 | 22 | 42 | 83 |
EDA-Descriptive Statistics¶
Basics¶
df_sample.info()
<class 'pandas.core.frame.DataFrame'>
Int64Index: 100000 entries, 24636210 to 1792721
Data columns (total 24 columns):
# Column Non-Null Count Dtype
--- ------ -------------- -----
0 date_time 100000 non-null datetime64[ns]
1 site_name 100000 non-null int64
2 posa_continent 100000 non-null int64
3 user_location_country 100000 non-null int64
4 user_location_region 100000 non-null int64
5 user_location_city 100000 non-null int64
6 orig_destination_distance 63915 non-null float64
7 user_id 100000 non-null int64
8 is_mobile 100000 non-null int64
9 is_package 100000 non-null int64
10 channel 100000 non-null int64
11 srch_ci 99878 non-null datetime64[ns]
12 srch_co 99878 non-null datetime64[ns]
13 srch_adults_cnt 100000 non-null int64
14 srch_children_cnt 100000 non-null int64
15 srch_rm_cnt 100000 non-null int64
16 srch_destination_id 100000 non-null int64
17 srch_destination_type_id 100000 non-null int64
18 is_booking 100000 non-null int64
19 cnt 100000 non-null int64
20 hotel_continent 100000 non-null int64
21 hotel_country 100000 non-null int64
22 hotel_market 100000 non-null int64
23 hotel_cluster 100000 non-null int64
dtypes: datetime64[ns](3), float64(1), int64(20)
memory usage: 19.1 MB
Attention
Almost a third of
orig_destination_distance
is missingSome rows are missing in srch_ci & srch_co. [~122]
df_sample.describe().T
count | mean | std | min | 25% | 50% | 75% | max | |
---|---|---|---|---|---|---|---|---|
site_name | 100000.0 | 9.838570 | 11.996426 | 2.0000 | 2.0000 | 2.0000 | 15.00000 | 5.300000e+01 |
posa_continent | 100000.0 | 2.678150 | 0.749018 | 0.0000 | 3.0000 | 3.0000 | 3.00000 | 4.000000e+00 |
user_location_country | 100000.0 | 85.995070 | 59.292002 | 0.0000 | 66.0000 | 66.0000 | 70.00000 | 2.390000e+02 |
user_location_region | 100000.0 | 308.636990 | 208.706581 | 0.0000 | 174.0000 | 314.0000 | 385.00000 | 1.025000e+03 |
user_location_city | 100000.0 | 27676.391240 | 16768.647409 | 0.0000 | 12949.0000 | 27655.0000 | 42328.00000 | 5.650700e+04 |
orig_destination_distance | 63915.0 | 1960.661721 | 2228.153377 | 0.0056 | 312.7921 | 1131.8353 | 2542.35935 | 1.196538e+04 |
user_id | 100000.0 | 605438.332130 | 351615.377136 | 14.0000 | 298796.2500 | 602882.5000 | 915722.25000 | 1.198783e+06 |
is_mobile | 100000.0 | 0.133770 | 0.340407 | 0.0000 | 0.0000 | 0.0000 | 0.00000 | 1.000000e+00 |
is_package | 100000.0 | 0.248140 | 0.431936 | 0.0000 | 0.0000 | 0.0000 | 0.00000 | 1.000000e+00 |
channel | 100000.0 | 5.858590 | 3.722823 | 0.0000 | 2.0000 | 9.0000 | 9.00000 | 1.000000e+01 |
srch_adults_cnt | 100000.0 | 2.020710 | 0.909774 | 0.0000 | 2.0000 | 2.0000 | 2.00000 | 9.000000e+00 |
srch_children_cnt | 100000.0 | 0.336750 | 0.734449 | 0.0000 | 0.0000 | 0.0000 | 0.00000 | 9.000000e+00 |
srch_rm_cnt | 100000.0 | 1.111750 | 0.451602 | 0.0000 | 1.0000 | 1.0000 | 1.00000 | 8.000000e+00 |
srch_destination_id | 100000.0 | 14426.327380 | 11036.981220 | 4.0000 | 8267.0000 | 9145.0000 | 18784.00000 | 6.503500e+04 |
srch_destination_type_id | 100000.0 | 2.577290 | 2.149263 | 1.0000 | 1.0000 | 1.0000 | 5.00000 | 9.000000e+00 |
is_booking | 100000.0 | 0.079930 | 0.271186 | 0.0000 | 0.0000 | 0.0000 | 0.00000 | 1.000000e+00 |
cnt | 100000.0 | 1.487980 | 1.280262 | 1.0000 | 1.0000 | 1.0000 | 2.00000 | 1.200000e+02 |
hotel_continent | 100000.0 | 3.150920 | 1.620534 | 0.0000 | 2.0000 | 2.0000 | 4.00000 | 6.000000e+00 |
hotel_country | 100000.0 | 81.093290 | 56.111519 | 0.0000 | 50.0000 | 50.0000 | 106.00000 | 2.120000e+02 |
hotel_market | 100000.0 | 598.848330 | 510.959297 | 0.0000 | 158.0000 | 591.0000 | 701.00000 | 2.117000e+03 |
hotel_cluster | 100000.0 | 49.860370 | 28.885423 | 0.0000 | 25.0000 | 49.0000 | 73.00000 | 9.900000e+01 |
df_sample['user_location_region'].nunique()
779
df_sample['user_location_city'].nunique()
10779
df_sample['date_time'].min(), df_sample['date_time'].max()
(Timestamp('2013-01-07 00:33:47'), Timestamp('2014-12-31 23:47:14'))
df_sample['srch_ci'].min(), df_sample['srch_ci'].max()
(Timestamp('2013-01-07 00:00:00'), Timestamp('2016-05-10 00:00:00'))
df_sample['srch_co'].min(), df_sample['srch_co'].max()
(Timestamp('2013-01-08 00:00:00'), Timestamp('2016-05-13 00:00:00'))
df_sample.nunique()
date_time 99886
site_name 41
posa_continent 5
user_location_country 201
user_location_region 779
user_location_city 10779
orig_destination_distance 62206
user_id 88863
is_mobile 2
is_package 2
channel 11
srch_ci 1066
srch_co 1068
srch_adults_cnt 10
srch_children_cnt 10
srch_rm_cnt 9
srch_destination_id 8827
srch_destination_type_id 8
is_booking 2
cnt 32
hotel_continent 7
hotel_country 178
hotel_market 1843
hotel_cluster 100
dtype: int64
Correlation¶
Correlation between booking and hotel room
pd.crosstab(df_sample['is_booking'], df_sample['srch_rm_cnt'])
srch_rm_cnt | 0 | 1 | 2 | 3 | 4 | 5 | 6 | 7 | 8 |
---|---|---|---|---|---|---|---|---|---|
is_booking | |||||||||
0 | 0 | 84425 | 6091 | 975 | 253 | 108 | 61 | 29 | 65 |
1 | 1 | 7248 | 580 | 102 | 42 | 9 | 6 | 1 | 4 |
df_sample.groupby('srch_rm_cnt')['is_booking'].mean()
srch_rm_cnt
0 1.000000
1 0.079064
2 0.086943
3 0.094708
4 0.142373
5 0.076923
6 0.089552
7 0.033333
8 0.057971
Name: is_booking, dtype: float64
df_sample.groupby('srch_rm_cnt')['is_booking'].value_counts()
srch_rm_cnt is_booking
0 1 1
1 0 84425
1 7248
2 0 6091
1 580
3 0 975
1 102
4 0 253
1 42
5 0 108
1 9
6 0 61
1 6
7 0 29
1 1
8 0 65
1 4
Name: is_booking, dtype: int64
df_sample.groupby('srch_rm_cnt')['is_booking'].mean()
srch_rm_cnt
0 1.000000
1 0.079064
2 0.086943
3 0.094708
4 0.142373
5 0.076923
6 0.089552
7 0.033333
8 0.057971
Name: is_booking, dtype: float64
df_sample.corr()
site_name | posa_continent | user_location_country | user_location_region | user_location_city | orig_destination_distance | user_id | is_mobile | is_package | channel | ... | srch_children_cnt | srch_rm_cnt | srch_destination_id | srch_destination_type_id | is_booking | cnt | hotel_continent | hotel_country | hotel_market | hotel_cluster | |
---|---|---|---|---|---|---|---|---|---|---|---|---|---|---|---|---|---|---|---|---|---|
site_name | 1.000000 | -0.637743 | 0.159283 | 0.130818 | -0.013471 | 0.027609 | 0.030404 | -0.005418 | 0.048820 | -0.027780 | ... | -0.031962 | 0.016585 | 0.034895 | -0.006934 | -0.013460 | 0.022274 | 0.201760 | 0.263167 | -0.068316 | -0.026689 |
posa_continent | -0.637743 | 1.000000 | 0.179726 | -0.034647 | 0.039227 | 0.049808 | -0.015209 | 0.016331 | -0.093459 | 0.089680 | ... | 0.034453 | -0.033712 | -0.015535 | 0.037172 | 0.013319 | -0.018952 | -0.333578 | -0.156578 | 0.049214 | 0.018297 |
user_location_country | 0.159283 | 0.179726 | 1.000000 | 0.058496 | 0.122686 | 0.047689 | -0.021091 | 0.003728 | -0.025284 | 0.109999 | ... | 0.037101 | 0.000858 | 0.013486 | 0.028888 | 0.001284 | 0.003539 | -0.063744 | 0.097624 | 0.015569 | -0.011876 |
user_location_region | 0.130818 | -0.034647 | 0.058496 | 1.000000 | 0.132457 | 0.136560 | 0.002225 | 0.016982 | 0.040482 | -0.001600 | ... | 0.014009 | 0.000254 | 0.022567 | 0.001376 | 0.000253 | -0.007570 | 0.043027 | -0.050301 | 0.040367 | 0.004984 |
user_location_city | -0.013471 | 0.039227 | 0.122686 | 0.132457 | 1.000000 | 0.014178 | -0.007989 | -0.003741 | 0.013032 | 0.023497 | ... | 0.002638 | -0.000694 | 0.000786 | -0.004399 | -0.002655 | -0.002175 | 0.007759 | -0.001987 | 0.008558 | 0.000102 |
orig_destination_distance | 0.027609 | 0.049808 | 0.047689 | 0.136560 | 0.014178 | 1.000000 | 0.017015 | -0.059464 | 0.041991 | -0.000398 | ... | -0.059722 | -0.012484 | -0.036314 | -0.042859 | -0.033480 | 0.009483 | 0.416180 | 0.254321 | -0.090112 | 0.003624 |
user_id | 0.030404 | -0.015209 | -0.021091 | 0.002225 | -0.007989 | 0.017015 | 1.000000 | -0.011439 | -0.018901 | -0.003593 | ... | 0.002983 | -0.001625 | 0.002716 | 0.007133 | 0.001561 | 0.001355 | 0.002447 | 0.008707 | -0.002463 | 0.003202 |
is_mobile | -0.005418 | 0.016331 | 0.003728 | 0.016982 | -0.003741 | -0.059464 | -0.011439 | 1.000000 | 0.046903 | -0.030770 | ... | 0.018211 | -0.022565 | -0.007140 | -0.016039 | -0.028623 | 0.008084 | -0.024144 | -0.029574 | 0.007644 | 0.012145 |
is_package | 0.048820 | -0.093459 | -0.025284 | 0.040482 | 0.013032 | 0.041991 | -0.018901 | 0.046903 | 1.000000 | -0.011269 | ... | -0.037673 | -0.036653 | -0.146647 | -0.224422 | -0.081307 | 0.126500 | 0.108993 | -0.044426 | -0.014636 | 0.031399 |
channel | -0.027780 | 0.089680 | 0.109999 | -0.001600 | 0.023497 | -0.000398 | -0.003593 | -0.030770 | -0.011269 | 1.000000 | ... | 0.004202 | 0.010191 | -0.000392 | 0.021612 | 0.025697 | -0.010248 | -0.022241 | -0.001217 | 0.006164 | 0.002596 |
srch_adults_cnt | -0.013405 | 0.012350 | 0.042526 | 0.005487 | 0.006628 | -0.024039 | -0.007370 | 0.016661 | -0.024097 | -0.014931 | ... | 0.107061 | 0.525970 | 0.005651 | -0.012119 | -0.046350 | 0.014024 | -0.019355 | -0.018169 | 0.010203 | 0.006482 |
srch_children_cnt | -0.031962 | 0.034453 | 0.037101 | 0.014009 | 0.002638 | -0.059722 | 0.002983 | 0.018211 | -0.037673 | 0.004202 | ... | 1.000000 | 0.091711 | -0.008784 | -0.007217 | -0.023228 | 0.019242 | -0.061707 | -0.045921 | 0.005056 | 0.021477 |
srch_rm_cnt | 0.016585 | -0.033712 | 0.000858 | 0.000254 | -0.000694 | -0.012484 | -0.001625 | -0.022565 | -0.036653 | 0.010191 | ... | 0.091711 | 1.000000 | 0.018139 | 0.013618 | 0.009454 | -0.000487 | 0.019150 | 0.011055 | 0.000104 | -0.012177 |
srch_destination_id | 0.034895 | -0.015535 | 0.013486 | 0.022567 | 0.000786 | -0.036314 | 0.002716 | -0.007140 | -0.146647 | -0.000392 | ... | -0.008784 | 0.018139 | 1.000000 | 0.435605 | 0.027674 | -0.021947 | 0.030365 | 0.053862 | 0.081240 | -0.010406 |
srch_destination_type_id | -0.006934 | 0.037172 | 0.028888 | 0.001376 | -0.004399 | -0.042859 | 0.007133 | -0.016039 | -0.224422 | 0.021612 | ... | -0.007217 | 0.013618 | 0.435605 | 1.000000 | 0.037398 | -0.024544 | -0.035655 | -0.021522 | 0.035783 | -0.033039 |
is_booking | -0.013460 | 0.013319 | 0.001284 | 0.000253 | -0.002655 | -0.033480 | 0.001561 | -0.028623 | -0.081307 | 0.025697 | ... | -0.023228 | 0.009454 | 0.027674 | 0.037398 | 1.000000 | -0.108628 | -0.025629 | -0.004763 | 0.012633 | -0.018192 |
cnt | 0.022274 | -0.018952 | 0.003539 | -0.007570 | -0.002175 | 0.009483 | 0.001355 | 0.008084 | 0.126500 | -0.010248 | ... | 0.019242 | -0.000487 | -0.021947 | -0.024544 | -0.108628 | 1.000000 | 0.020670 | 0.001443 | -0.008747 | -0.000607 |
hotel_continent | 0.201760 | -0.333578 | -0.063744 | 0.043027 | 0.007759 | 0.416180 | 0.002447 | -0.024144 | 0.108993 | -0.022241 | ... | -0.061707 | 0.019150 | 0.030365 | -0.035655 | -0.025629 | 0.020670 | 1.000000 | 0.295991 | -0.096278 | -0.015632 |
hotel_country | 0.263167 | -0.156578 | 0.097624 | -0.050301 | -0.001987 | 0.254321 | 0.008707 | -0.029574 | -0.044426 | -0.001217 | ... | -0.045921 | 0.011055 | 0.053862 | -0.021522 | -0.004763 | 0.001443 | 0.295991 | 1.000000 | 0.017868 | -0.025002 |
hotel_market | -0.068316 | 0.049214 | 0.015569 | 0.040367 | 0.008558 | -0.090112 | -0.002463 | 0.007644 | -0.014636 | 0.006164 | ... | 0.005056 | 0.000104 | 0.081240 | 0.035783 | 0.012633 | -0.008747 | -0.096278 | 0.017868 | 1.000000 | 0.037060 |
hotel_cluster | -0.026689 | 0.018297 | -0.011876 | 0.004984 | 0.000102 | 0.003624 | 0.003202 | 0.012145 | 0.031399 | 0.002596 | ... | 0.021477 | -0.012177 | -0.010406 | -0.033039 | -0.018192 | -0.000607 | -0.015632 | -0.025002 | 0.037060 | 1.000000 |
21 rows × 21 columns
fig, ax = plt.subplots(figsize=(12,12))
sns.heatmap(df_sample.corr(),ax =ax)
plt.show()
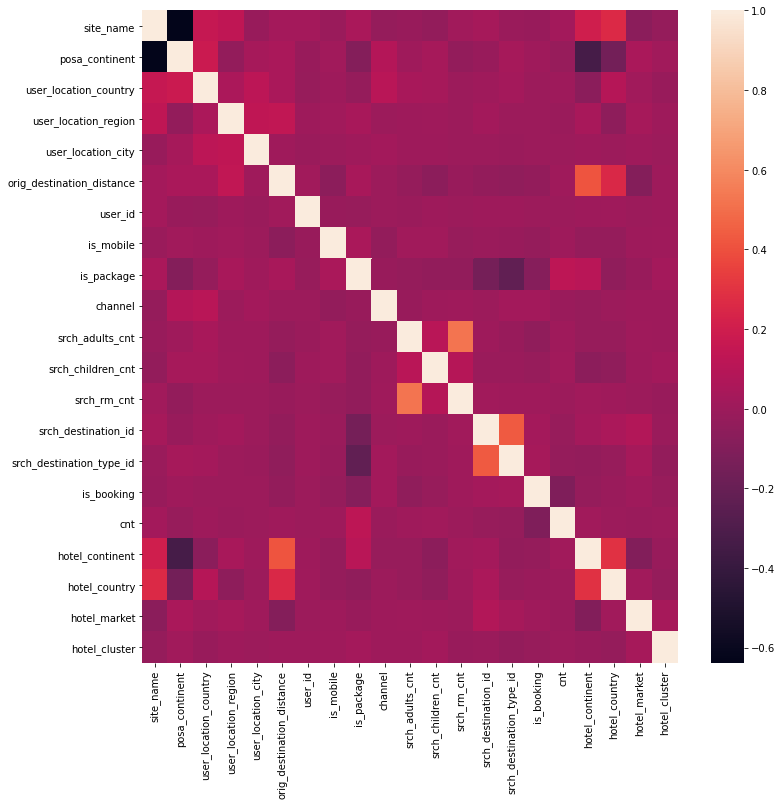
df_sample['srch_children_cnt'].corr(df_sample['is_booking'])
-0.023228499097508428
sns.pairplot(df_sample)
<seaborn.axisgrid.PairGrid at 0x7f34f56e0070>
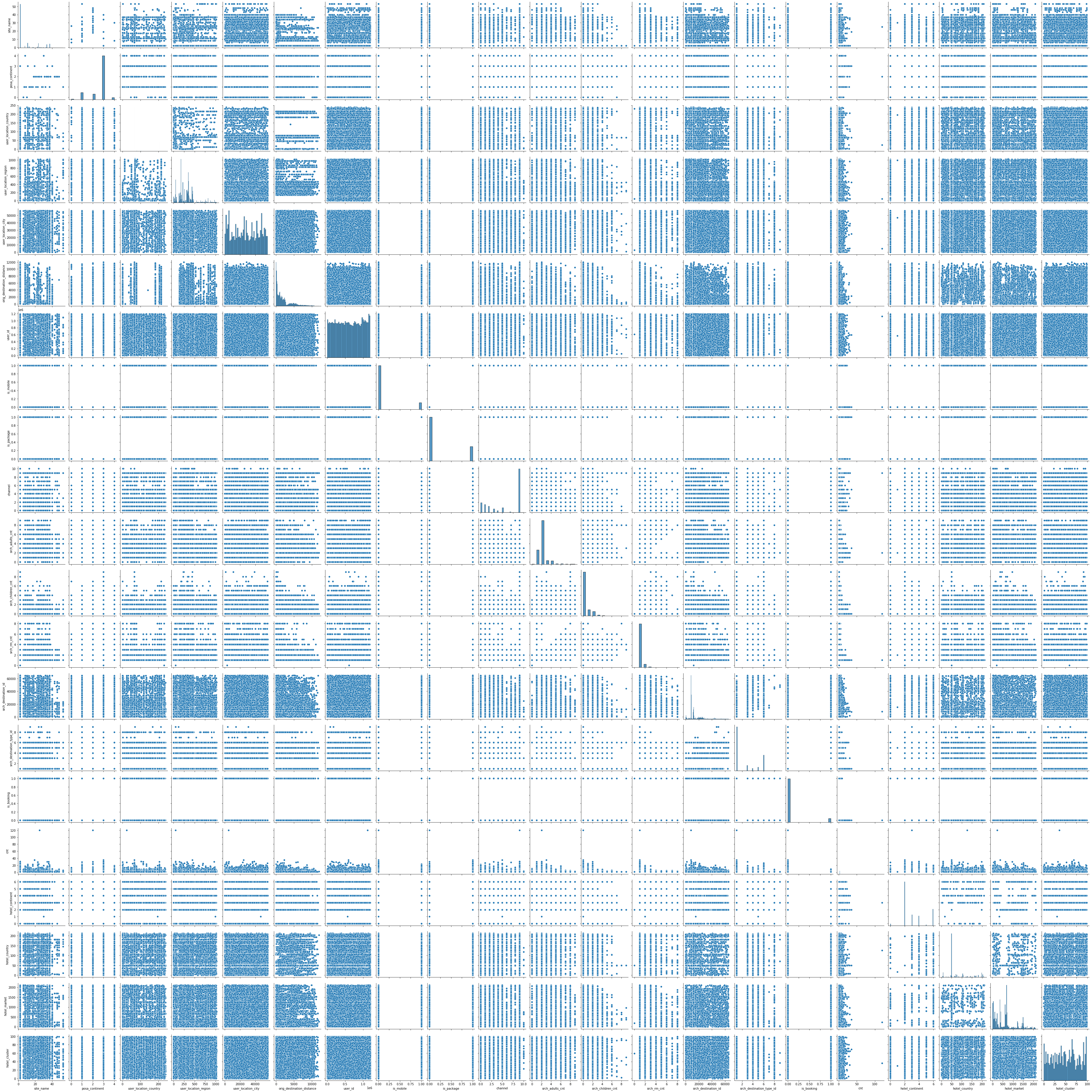
selection = ['channel', 'is_booking', 'is_mobile', 'orig_destination_distance', 'srch_rm_cnt', 'srch_adults_cnt', 'srch_children_cnt']
fig, axes = plt.subplots(nrows=1, ncols=1, figsize=(12,12)); axes
ax1 = axes
sns.heatmap(df_sample[selection].corr(),ax =ax1)
plt.tight_layout()
plt.show()
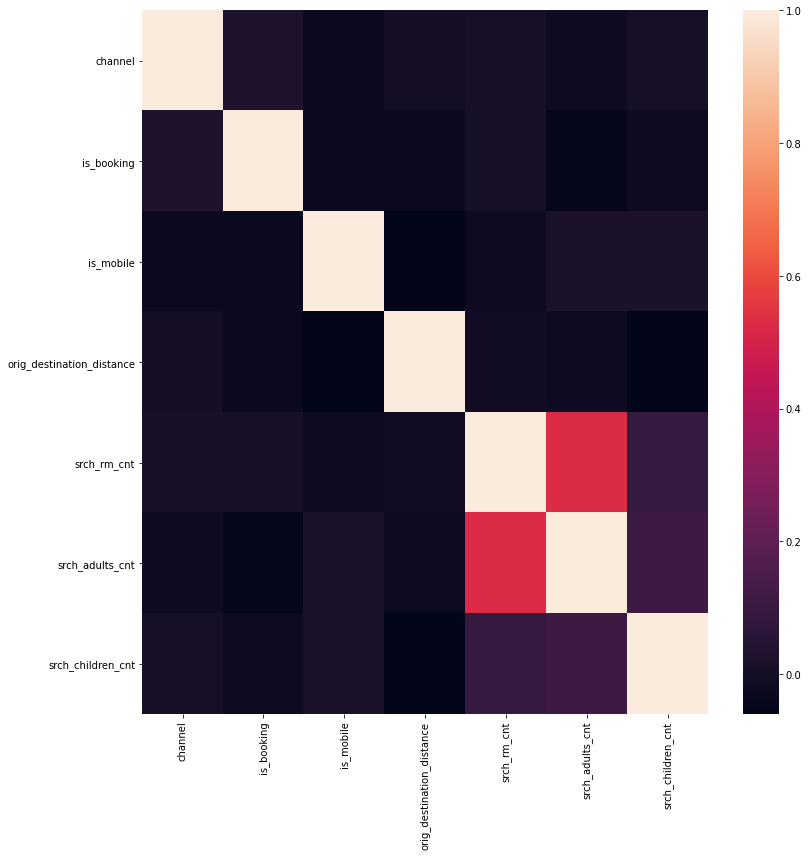
sns.pairplot(df_sample[selection], hue="is_booking", height=2.5)
<seaborn.axisgrid.PairGrid at 0x7f33e88640a0>
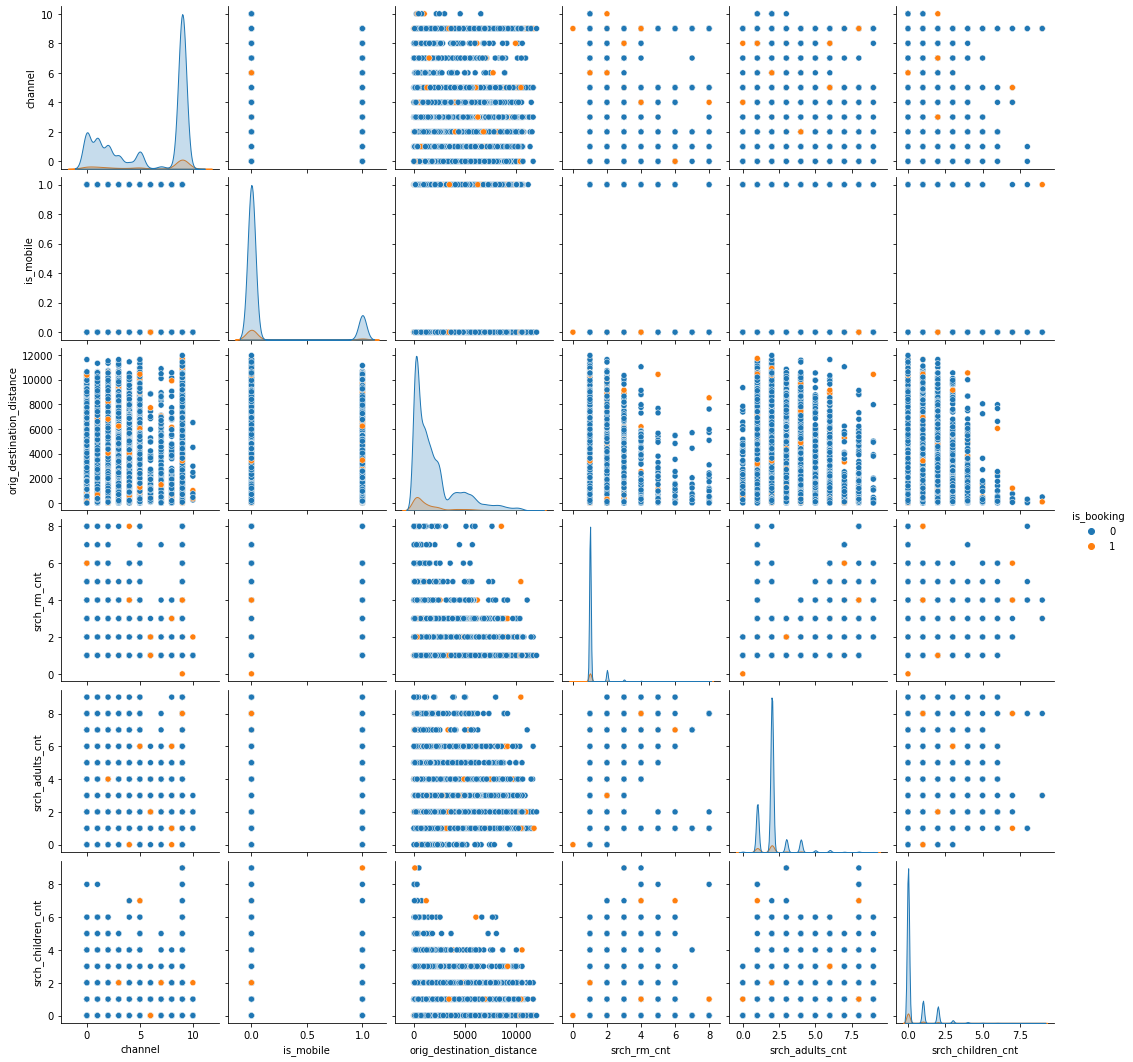
fig, ax = plt.subplots(1,1,figsize=(12,12))
df_sample[selection].hist(ax=fig.gca())
plt.show()
<ipython-input-73-88919a001020>:2: UserWarning: To output multiple subplots, the figure containing the passed axes is being cleared
df_sample[selection].hist(ax=fig.gca())
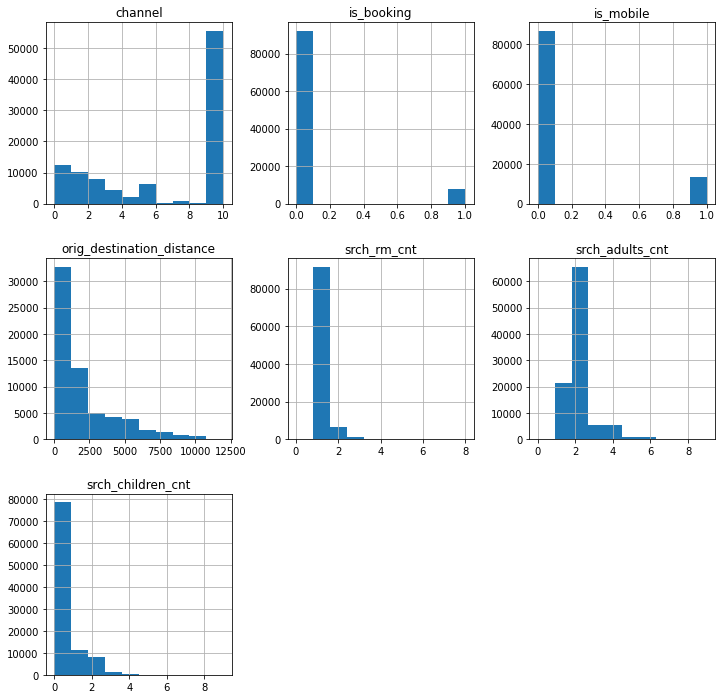
# sns.kdeplot(df_sample[selection])
Distribution of number of Booking Attempts¶
df_userbooking = df_sample.groupby('user_id')['is_booking']\
.agg(number_of_booking='count').reset_index()
df_userbooking
user_id | number_of_booking | |
---|---|---|
0 | 14 | 1 |
1 | 38 | 1 |
2 | 40 | 1 |
3 | 156 | 2 |
4 | 160 | 1 |
... | ... | ... |
88858 | 1198722 | 1 |
88859 | 1198742 | 1 |
88860 | 1198748 | 1 |
88861 | 1198776 | 1 |
88862 | 1198783 | 2 |
88863 rows × 2 columns
df_sample = df_sample.merge(df_userbooking)
df_sample.head().T
0 | 1 | 2 | 3 | 4 | |
---|---|---|---|---|---|
date_time | 2014-11-03 16:02:28 | 2014-07-28 23:50:54 | 2013-03-13 19:25:01 | 2014-10-13 13:20:25 | 2013-11-05 10:40:34 |
site_name | 24 | 24 | 11 | 2 | 11 |
posa_continent | 2 | 2 | 3 | 3 | 3 |
user_location_country | 77 | 77 | 205 | 66 | 205 |
user_location_region | 871 | 871 | 135 | 314 | 411 |
user_location_city | 36643 | 36643 | 38749 | 48562 | 52752 |
orig_destination_distance | 456.115 | 454.461 | 232.474 | 4468.27 | 171.602 |
user_id | 792280 | 792280 | 961995 | 495669 | 106611 |
is_mobile | 0 | 0 | 0 | 0 | 0 |
is_package | 1 | 1 | 0 | 1 | 0 |
channel | 1 | 9 | 9 | 9 | 0 |
srch_ci | 2014-12-15 00:00:00 | 2014-08-26 00:00:00 | 2013-03-13 00:00:00 | 2015-04-03 00:00:00 | 2013-11-07 00:00:00 |
srch_co | 2014-12-19 00:00:00 | 2014-08-31 00:00:00 | 2013-03-14 00:00:00 | 2015-04-10 00:00:00 | 2013-11-08 00:00:00 |
srch_adults_cnt | 2 | 1 | 2 | 2 | 2 |
srch_children_cnt | 0 | 0 | 0 | 0 | 0 |
srch_rm_cnt | 1 | 1 | 1 | 1 | 1 |
srch_destination_id | 8286 | 8286 | 1842 | 8746 | 6210 |
srch_destination_type_id | 1 | 1 | 3 | 1 | 3 |
is_booking | 0 | 0 | 0 | 0 | 1 |
cnt | 1 | 1 | 1 | 1 | 1 |
hotel_continent | 0 | 0 | 2 | 6 | 2 |
hotel_country | 63 | 63 | 198 | 105 | 198 |
hotel_market | 1258 | 1258 | 786 | 29 | 1234 |
hotel_cluster | 68 | 14 | 37 | 22 | 42 |
number_of_booking | 2 | 2 | 1 | 1 | 2 |
df_sample.groupby('user_id')['is_booking']\
.agg(['mean']).reset_index()\
.groupby('mean')['user_id']\
.agg('count')
mean
0.000000 80991
0.200000 5
0.250000 35
0.333333 153
0.400000 1
0.500000 1054
0.600000 1
0.666667 26
0.750000 1
1.000000 6596
Name: user_id, dtype: int64
(df_sample.groupby('user_id')['is_booking']\
.agg(['mean']).reset_index()\
.groupby('mean')['user_id']\
.agg('count')).plot(x='mean', y='user_id')
plt.show()
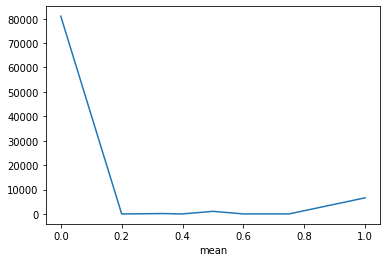
EDA-Business Rules Validation¶
Remove columns with zero occupant¶
pd.crosstab(df_sample['srch_adults_cnt'], df_sample['srch_children_cnt'])
srch_children_cnt | 0 | 1 | 2 | 3 | 4 | 5 | 6 | 7 | 8 | 9 |
---|---|---|---|---|---|---|---|---|---|---|
srch_adults_cnt | ||||||||||
0 | 174 | 2 | 3 | 2 | 0 | 0 | 0 | 0 | 0 | 0 |
1 | 18749 | 2137 | 523 | 117 | 11 | 1 | 9 | 1 | 2 | 0 |
2 | 50736 | 7093 | 6529 | 972 | 208 | 14 | 7 | 1 | 0 | 0 |
3 | 3645 | 1131 | 469 | 131 | 27 | 5 | 2 | 2 | 0 | 2 |
4 | 3933 | 690 | 494 | 77 | 83 | 9 | 4 | 0 | 0 | 0 |
5 | 535 | 131 | 41 | 20 | 6 | 4 | 2 | 0 | 0 | 0 |
6 | 669 | 73 | 53 | 28 | 18 | 13 | 7 | 0 | 0 | 0 |
7 | 99 | 20 | 5 | 8 | 6 | 3 | 0 | 0 | 0 | 0 |
8 | 183 | 12 | 13 | 2 | 6 | 1 | 3 | 2 | 2 | 1 |
9 | 24 | 5 | 4 | 2 | 1 | 1 | 2 | 0 | 0 | 0 |
(df_sample['srch_adults_cnt']+df_sample['srch_children_cnt']) == 0
0 False
1 False
2 False
3 False
4 False
...
99995 False
99996 False
99997 False
99998 False
99999 False
Length: 100000, dtype: bool
df_sample = df_sample[(df_sample['srch_adults_cnt']+df_sample['srch_children_cnt']) > 0]
df_sample
date_time | site_name | posa_continent | user_location_country | user_location_region | user_location_city | orig_destination_distance | user_id | is_mobile | is_package | ... | srch_rm_cnt | srch_destination_id | srch_destination_type_id | is_booking | cnt | hotel_continent | hotel_country | hotel_market | hotel_cluster | number_of_booking | |
---|---|---|---|---|---|---|---|---|---|---|---|---|---|---|---|---|---|---|---|---|---|
0 | 2014-11-03 16:02:28 | 24 | 2 | 77 | 871 | 36643 | 456.1151 | 792280 | 0 | 1 | ... | 1 | 8286 | 1 | 0 | 1 | 0 | 63 | 1258 | 68 | 2 |
1 | 2014-07-28 23:50:54 | 24 | 2 | 77 | 871 | 36643 | 454.4611 | 792280 | 0 | 1 | ... | 1 | 8286 | 1 | 0 | 1 | 0 | 63 | 1258 | 14 | 2 |
2 | 2013-03-13 19:25:01 | 11 | 3 | 205 | 135 | 38749 | 232.4737 | 961995 | 0 | 0 | ... | 1 | 1842 | 3 | 0 | 1 | 2 | 198 | 786 | 37 | 1 |
3 | 2014-10-13 13:20:25 | 2 | 3 | 66 | 314 | 48562 | 4468.2720 | 495669 | 0 | 1 | ... | 1 | 8746 | 1 | 0 | 1 | 6 | 105 | 29 | 22 | 1 |
4 | 2013-11-05 10:40:34 | 11 | 3 | 205 | 411 | 52752 | 171.6021 | 106611 | 0 | 0 | ... | 1 | 6210 | 3 | 1 | 1 | 2 | 198 | 1234 | 42 | 2 |
... | ... | ... | ... | ... | ... | ... | ... | ... | ... | ... | ... | ... | ... | ... | ... | ... | ... | ... | ... | ... | ... |
99995 | 2013-03-31 16:45:01 | 2 | 3 | 66 | 351 | 21609 | 1386.4061 | 858268 | 0 | 0 | ... | 1 | 762 | 6 | 0 | 1 | 2 | 50 | 503 | 91 | 1 |
99996 | 2013-09-29 14:09:07 | 2 | 3 | 66 | 462 | 49272 | 698.1395 | 957708 | 0 | 0 | ... | 1 | 12843 | 5 | 0 | 1 | 2 | 50 | 661 | 6 | 1 |
99997 | 2014-07-11 22:05:54 | 37 | 1 | 69 | 998 | 52849 | NaN | 814512 | 1 | 1 | ... | 3 | 18773 | 1 | 0 | 1 | 6 | 22 | 1794 | 38 | 1 |
99998 | 2013-07-02 01:03:12 | 2 | 3 | 66 | 246 | 28491 | 207.2549 | 881704 | 0 | 1 | ... | 1 | 8859 | 1 | 0 | 1 | 2 | 50 | 212 | 89 | 1 |
99999 | 2014-12-19 19:59:12 | 11 | 3 | 205 | 354 | 53478 | 1198.4359 | 845482 | 0 | 0 | ... | 1 | 11848 | 1 | 0 | 1 | 2 | 50 | 705 | 42 | 1 |
99826 rows × 25 columns
Create a date column¶
df_sample.loc[:,'date'] =df_sample['date_time'].dt.date
df_sample.head().T
0 | 1 | 2 | 3 | 4 | |
---|---|---|---|---|---|
date_time | 2014-11-03 16:02:28 | 2014-07-28 23:50:54 | 2013-03-13 19:25:01 | 2014-10-13 13:20:25 | 2013-11-05 10:40:34 |
site_name | 24 | 24 | 11 | 2 | 11 |
posa_continent | 2 | 2 | 3 | 3 | 3 |
user_location_country | 77 | 77 | 205 | 66 | 205 |
user_location_region | 871 | 871 | 135 | 314 | 411 |
user_location_city | 36643 | 36643 | 38749 | 48562 | 52752 |
orig_destination_distance | 456.115 | 454.461 | 232.474 | 4468.27 | 171.602 |
user_id | 792280 | 792280 | 961995 | 495669 | 106611 |
is_mobile | 0 | 0 | 0 | 0 | 0 |
is_package | 1 | 1 | 0 | 1 | 0 |
channel | 1 | 9 | 9 | 9 | 0 |
srch_ci | 2014-12-15 00:00:00 | 2014-08-26 00:00:00 | 2013-03-13 00:00:00 | 2015-04-03 00:00:00 | 2013-11-07 00:00:00 |
srch_co | 2014-12-19 00:00:00 | 2014-08-31 00:00:00 | 2013-03-14 00:00:00 | 2015-04-10 00:00:00 | 2013-11-08 00:00:00 |
srch_adults_cnt | 2 | 1 | 2 | 2 | 2 |
srch_children_cnt | 0 | 0 | 0 | 0 | 0 |
srch_rm_cnt | 1 | 1 | 1 | 1 | 1 |
srch_destination_id | 8286 | 8286 | 1842 | 8746 | 6210 |
srch_destination_type_id | 1 | 1 | 3 | 1 | 3 |
is_booking | 0 | 0 | 0 | 0 | 1 |
cnt | 1 | 1 | 1 | 1 | 1 |
hotel_continent | 0 | 0 | 2 | 6 | 2 |
hotel_country | 63 | 63 | 198 | 105 | 198 |
hotel_market | 1258 | 1258 | 786 | 29 | 1234 |
hotel_cluster | 68 | 14 | 37 | 22 | 42 |
number_of_booking | 2 | 2 | 1 | 1 | 2 |
date | 2014-11-03 | 2014-07-28 | 2013-03-13 | 2014-10-13 | 2013-11-05 |
df_sample.shape
(99826, 26)
Check booking date < check_in date < check out date¶
chk = df_sample[(df_sample['srch_ci']<df_sample['date']) |
(df_sample['srch_co'] <df_sample['srch_ci'])]
chk.shape
(27, 26)
EDA - Create New Features¶
# duration
df_sample['duration'] = (df_sample['srch_co'] - df_sample['srch_ci'])/ np.timedelta64(1, "D")
df_sample['duration']
0 4.0
1 5.0
2 1.0
3 7.0
4 1.0
...
99995 4.0
99996 1.0
99997 7.0
99998 2.0
99999 3.0
Name: duration, Length: 99826, dtype: float64
df_sample.loc[df_sample['duration'] < 0, 'duration'] = np.nan
df_sample['days_in_advance'] = (df_sample['srch_ci'] - df_sample['date_time'])/ np.timedelta64(1, "D")
df_sample.loc[df_sample['days_in_advance'] < 0, 'days_in_advance'] = np.nan
df_sample.head().T
0 | 1 | 2 | 3 | 4 | |
---|---|---|---|---|---|
date_time | 2014-11-03 16:02:28 | 2014-07-28 23:50:54 | 2013-03-13 19:25:01 | 2014-10-13 13:20:25 | 2013-11-05 10:40:34 |
site_name | 24 | 24 | 11 | 2 | 11 |
posa_continent | 2 | 2 | 3 | 3 | 3 |
user_location_country | 77 | 77 | 205 | 66 | 205 |
user_location_region | 871 | 871 | 135 | 314 | 411 |
user_location_city | 36643 | 36643 | 38749 | 48562 | 52752 |
orig_destination_distance | 456.115 | 454.461 | 232.474 | 4468.27 | 171.602 |
user_id | 792280 | 792280 | 961995 | 495669 | 106611 |
is_mobile | 0 | 0 | 0 | 0 | 0 |
is_package | 1 | 1 | 0 | 1 | 0 |
channel | 1 | 9 | 9 | 9 | 0 |
srch_ci | 2014-12-15 00:00:00 | 2014-08-26 00:00:00 | 2013-03-13 00:00:00 | 2015-04-03 00:00:00 | 2013-11-07 00:00:00 |
srch_co | 2014-12-19 00:00:00 | 2014-08-31 00:00:00 | 2013-03-14 00:00:00 | 2015-04-10 00:00:00 | 2013-11-08 00:00:00 |
srch_adults_cnt | 2 | 1 | 2 | 2 | 2 |
srch_children_cnt | 0 | 0 | 0 | 0 | 0 |
srch_rm_cnt | 1 | 1 | 1 | 1 | 1 |
srch_destination_id | 8286 | 8286 | 1842 | 8746 | 6210 |
srch_destination_type_id | 1 | 1 | 3 | 1 | 3 |
is_booking | 0 | 0 | 0 | 0 | 1 |
cnt | 1 | 1 | 1 | 1 | 1 |
hotel_continent | 0 | 0 | 2 | 6 | 2 |
hotel_country | 63 | 63 | 198 | 105 | 198 |
hotel_market | 1258 | 1258 | 786 | 29 | 1234 |
hotel_cluster | 68 | 14 | 37 | 22 | 42 |
number_of_booking | 2 | 2 | 1 | 1 | 2 |
date | 2014-11-03 | 2014-07-28 | 2013-03-13 | 2014-10-13 | 2013-11-05 |
duration | 4 | 5 | 1 | 7 | 1 |
days_in_advance | 41.3316 | 28.0063 | NaN | 171.444 | 1.55516 |
Underperforming and Overperforming Segments¶
Effect Size and Comparing to Mean¶
Here we are taking booking_rate
per segment as our effect size.
df_sample.channel.nunique()
11
df_sample.columns
Index(['date_time', 'site_name', 'posa_continent', 'user_location_country',
'user_location_region', 'user_location_city',
'orig_destination_distance', 'user_id', 'is_mobile', 'is_package',
'channel', 'srch_ci', 'srch_co', 'srch_adults_cnt', 'srch_children_cnt',
'srch_rm_cnt', 'srch_destination_id', 'srch_destination_type_id',
'is_booking', 'cnt', 'hotel_continent', 'hotel_country', 'hotel_market',
'hotel_cluster', 'number_of_booking', 'date', 'duration',
'days_in_advance'],
dtype='object')
cat_list = ['site_name', 'posa_continent', 'user_location_country',
'user_location_region', 'user_location_city','channel',
'srch_destination_id', 'srch_destination_type_id',
'hotel_continent', 'hotel_country', 'hotel_market',
'hotel_cluster']
df_sample.groupby('channel')['is_booking']\
.agg(booking_rate='mean', num_of_bookings='sum')\
.reset_index()\
.sort_values(by='booking_rate')
channel | booking_rate | num_of_bookings | |
---|---|---|---|
7 | 7 | 0.043263 | 35 |
8 | 8 | 0.051852 | 14 |
3 | 3 | 0.060482 | 266 |
2 | 2 | 0.060583 | 474 |
6 | 6 | 0.068323 | 11 |
1 | 1 | 0.069568 | 713 |
0 | 0 | 0.072184 | 901 |
9 | 9 | 0.085365 | 4719 |
5 | 5 | 0.094533 | 581 |
4 | 4 | 0.120438 | 264 |
10 | 10 | 0.200000 | 3 |
@interact
def display_ranking(cat=cat_list):
display(df_sample.groupby(cat)['is_booking']\
.agg(booking_rate='mean', num_of_bookings='sum')\
.reset_index()\
.sort_values(by='booking_rate', ascending=False))
## Population Booking Rate
df_sample['is_booking'].mean()
0.07994911145392984
Note
Looking at the data above we can see 4,5,9, 10 performing more than the population mean. However we need to ask the question are they really significant or just by chance?
Two Sample T-Test¶
Samples coming from 2 different group
Check each group for inference condition
Randomness
Independence n*p <= 10 n(1-p) <= 10
Normal
Sampled with Replacement
Sample size <= 10%N ( population)
Assign a signifance and confidence level ( for calculaing z*)
Calculate Confidence interval
\(CI = \hat{p}_1 - \hat{p}_1 \pm z^*\sqrt{ \frac{\hat{p}_1(1-\hat{p}_1)}{n_1}+ \frac{\hat{p}_2(1-\hat{p}_2)}{n_2}} \)
Calculate pvalue & t-test
df_sample.groupby('channel')['is_booking']\
.agg(average='mean',
bookings='count').reset_index()
channel | average | bookings | |
---|---|---|---|
0 | 0 | 0.072184 | 12482 |
1 | 1 | 0.069568 | 10249 |
2 | 2 | 0.060583 | 7824 |
3 | 3 | 0.060482 | 4398 |
4 | 4 | 0.120438 | 2192 |
5 | 5 | 0.094533 | 6146 |
6 | 6 | 0.068323 | 161 |
7 | 7 | 0.043263 | 809 |
8 | 8 | 0.051852 | 270 |
9 | 9 | 0.085365 | 55280 |
10 | 10 | 0.200000 | 15 |
def stat_comparison(cat, df):
cat = df.groupby(cat)['is_booking']\
.agg(sub_average='mean',
sub_bookings='count')\
.reset_index()
cat['overall_average'] = df['is_booking'].mean()
cat['overall_bookings'] = df['is_booking'].count()
cat['rest_bookings'] = cat['overall_bookings']-cat['sub_bookings']
cat['rest_average'] = (cat['overall_bookings']*cat['overall_average']- cat['sub_bookings']*cat['sub_average'])/cat['rest_bookings']
cat['z_score'] = (cat['sub_average']-cat['rest_average'])/\
np.sqrt(cat['overall_average']*(1-cat['overall_average'])
*(1/cat['sub_bookings']+1/cat['rest_bookings']))
cat['prob'] = np.around(stats.norm.cdf(cat['z_score']), decimals=10)
def significant(x):
if x >0.9:
return 1
elif x < 0.1:
return -1
else:
return 0
cat['significant'] = cat['prob'].apply(significant)
return cat
stat_comparison('channel', df_sample)
channel | sub_average | sub_bookings | overall_average | overall_bookings | rest_bookings | rest_average | z_score | prob | significant | |
---|---|---|---|---|---|---|---|---|---|---|
0 | 0 | 0.072184 | 12482 | 0.079949 | 99826 | 87344 | 0.081059 | -3.419680 | 3.134747e-04 | -1 |
1 | 1 | 0.069568 | 10249 | 0.079949 | 99826 | 89577 | 0.081137 | -4.090773 | 2.149690e-05 | -1 |
2 | 2 | 0.060583 | 7824 | 0.079949 | 99826 | 92002 | 0.081596 | -6.579173 | 0.000000e+00 | -1 |
3 | 3 | 0.060482 | 4398 | 0.079949 | 99826 | 95428 | 0.080846 | -4.868548 | 5.621000e-07 | -1 |
4 | 4 | 0.120438 | 2192 | 0.079949 | 99826 | 97634 | 0.079040 | 7.067474 | 1.000000e+00 | 1 |
5 | 5 | 0.094533 | 6146 | 0.079949 | 99826 | 93680 | 0.078992 | 4.351672 | 9.999932e-01 | 1 |
6 | 6 | 0.068323 | 161 | 0.079949 | 99826 | 99665 | 0.079968 | -0.544360 | 2.930970e-01 | 0 |
7 | 7 | 0.043263 | 809 | 0.079949 | 99826 | 99017 | 0.080249 | -3.863018 | 5.599740e-05 | -1 |
8 | 8 | 0.051852 | 270 | 0.079949 | 99826 | 99556 | 0.080025 | -1.704595 | 4.413499e-02 | -1 |
9 | 9 | 0.085365 | 55280 | 0.079949 | 99826 | 44546 | 0.073228 | 7.028968 | 1.000000e+00 | 1 |
10 | 10 | 0.200000 | 15 | 0.079949 | 99826 | 99811 | 0.079931 | 1.714474 | 9.567791e-01 | 1 |
@interact
def review_stats(cat=cat_list):
return stat_comparison(cat, df_sample)
Note
From above we can find significantly affected channel / cities/ region
Clustering - What are similar user cities?¶
Step1. What are the features I am going to use? (that makes sense)¶
What features may distinguish cities based on business sense and exploratory analysis
# Numerical Features
df_sample.columns
Index(['date_time', 'site_name', 'posa_continent', 'user_location_country',
'user_location_region', 'user_location_city',
'orig_destination_distance', 'user_id', 'is_mobile', 'is_package',
'channel', 'srch_ci', 'srch_co', 'srch_adults_cnt', 'srch_children_cnt',
'srch_rm_cnt', 'srch_destination_id', 'srch_destination_type_id',
'is_booking', 'cnt', 'hotel_continent', 'hotel_country', 'hotel_market',
'hotel_cluster', 'number_of_booking', 'date', 'duration',
'days_in_advance'],
dtype='object')
num_list = ['orig_destination_distance', 'is_mobile', 'is_package',
'srch_adults_cnt', 'srch_children_cnt',
'srch_rm_cnt', 'duration',
'days_in_advance']
city_data = df_sample[['user_location_city']+num_list].dropna(axis=0)
city_data
user_location_city | orig_destination_distance | is_mobile | is_package | srch_adults_cnt | srch_children_cnt | srch_rm_cnt | duration | days_in_advance | |
---|---|---|---|---|---|---|---|---|---|
0 | 36643 | 456.1151 | 0 | 1 | 2 | 0 | 1 | 4.0 | 41.331620 |
1 | 36643 | 454.4611 | 0 | 1 | 1 | 0 | 1 | 5.0 | 28.006319 |
3 | 48562 | 4468.2720 | 0 | 1 | 2 | 0 | 1 | 7.0 | 171.444155 |
4 | 52752 | 171.6021 | 0 | 0 | 2 | 0 | 1 | 1.0 | 1.555162 |
5 | 54864 | 209.6633 | 0 | 0 | 1 | 0 | 1 | 3.0 | 6.484433 |
... | ... | ... | ... | ... | ... | ... | ... | ... | ... |
99993 | 36878 | 368.5449 | 0 | 0 | 1 | 0 | 1 | 1.0 | 9.359387 |
99995 | 21609 | 1386.4061 | 0 | 0 | 2 | 0 | 1 | 4.0 | 96.302072 |
99996 | 49272 | 698.1395 | 0 | 0 | 1 | 0 | 1 | 1.0 | 0.410336 |
99998 | 28491 | 207.2549 | 0 | 1 | 2 | 0 | 1 | 2.0 | 10.956111 |
99999 | 53478 | 1198.4359 | 0 | 0 | 2 | 1 | 1 | 3.0 | 11.167222 |
61554 rows × 9 columns
city_groups = city_data.groupby('user_location_city')\
.mean().reset_index().dropna(); city_groups
user_location_city | orig_destination_distance | is_mobile | is_package | srch_adults_cnt | srch_children_cnt | srch_rm_cnt | duration | days_in_advance | |
---|---|---|---|---|---|---|---|---|---|
0 | 0 | 2315.836250 | 0.000000 | 0.250000 | 1.750000 | 0.000000 | 1.000000 | 2.000000 | 77.959358 |
1 | 3 | 3451.384159 | 0.058824 | 0.294118 | 1.941176 | 0.470588 | 1.000000 | 4.294118 | 87.139739 |
2 | 7 | 5994.864000 | 0.000000 | 1.000000 | 2.000000 | 0.000000 | 1.000000 | 14.000000 | 57.287755 |
3 | 14 | 5342.819100 | 0.000000 | 0.000000 | 2.000000 | 0.750000 | 1.000000 | 7.000000 | 35.206548 |
4 | 21 | 2165.768900 | 0.000000 | 0.500000 | 1.500000 | 1.000000 | 1.000000 | 5.000000 | 30.946875 |
... | ... | ... | ... | ... | ... | ... | ... | ... | ... |
4480 | 56472 | 1394.624100 | 0.214286 | 0.357143 | 2.000000 | 0.285714 | 1.142857 | 3.285714 | 83.805879 |
4481 | 56488 | 5930.875650 | 0.000000 | 0.000000 | 1.500000 | 0.000000 | 1.000000 | 6.000000 | 130.960440 |
4482 | 56498 | 3288.597750 | 0.500000 | 0.500000 | 3.000000 | 1.000000 | 1.500000 | 4.500000 | 49.570741 |
4483 | 56505 | 1370.771600 | 1.000000 | 0.000000 | 2.000000 | 0.000000 | 1.000000 | 2.000000 | 22.968461 |
4484 | 56507 | 1941.016580 | 0.400000 | 0.600000 | 2.600000 | 0.400000 | 1.400000 | 5.000000 | 127.597354 |
4485 rows × 9 columns
Step2. Standardize the data¶
What is magnitude of data range ?
city_groups_std = city_groups.copy()
sc = StandardScaler()
city_groups_std.loc[:, num_list]= sc.fit_transform(city_groups_std[num_list])
city_groups_std
user_location_city | orig_destination_distance | is_mobile | is_package | srch_adults_cnt | srch_children_cnt | srch_rm_cnt | duration | days_in_advance | |
---|---|---|---|---|---|---|---|---|---|
0 | 0 | 0.295943 | -0.583534 | -0.030898 | -0.510201 | -0.684278 | -0.325406 | -0.676476 | 0.438680 |
1 | 3 | 0.997273 | -0.321107 | 0.112882 | -0.215089 | 0.183626 | -0.325406 | 0.417193 | 0.636132 |
2 | 7 | 2.568161 | -0.583534 | 2.413358 | -0.124285 | -0.684278 | -0.325406 | 5.044256 | -0.005927 |
3 | 14 | 2.165449 | -0.583534 | -0.845650 | -0.124285 | 0.698944 | -0.325406 | 1.707162 | -0.480852 |
4 | 21 | 0.203260 | -0.583534 | 0.783854 | -0.896118 | 1.160018 | -0.325406 | 0.753707 | -0.572470 |
... | ... | ... | ... | ... | ... | ... | ... | ... | ... |
4480 | 56472 | -0.273010 | 0.372450 | 0.318282 | -0.124285 | -0.157336 | 0.063454 | -0.063540 | 0.564427 |
4481 | 56488 | 2.528641 | -0.583534 | -0.845650 | -0.896118 | -0.684278 | -0.325406 | 1.230435 | 1.578633 |
4482 | 56498 | 0.896734 | 1.647094 | 0.783854 | 1.419380 | 1.160018 | 1.035602 | 0.515343 | -0.171906 |
4483 | 56505 | -0.287742 | 3.877723 | -0.845650 | -0.124285 | -0.684278 | -0.325406 | -0.676476 | -0.744070 |
4484 | 56507 | 0.064449 | 1.200969 | 1.109755 | 0.801914 | 0.053441 | 0.763400 | 0.753707 | 1.506299 |
4485 rows × 9 columns
km = KMeans(n_clusters=3, max_iter=300, random_state=None)
city_groups_std['cluster'] = km.fit_predict(city_groups_std[num_list])
city_groups_std
user_location_city | orig_destination_distance | is_mobile | is_package | srch_adults_cnt | srch_children_cnt | srch_rm_cnt | duration | days_in_advance | cluster | |
---|---|---|---|---|---|---|---|---|---|---|
0 | 0 | 0.295943 | -0.583534 | -0.030898 | -0.510201 | -0.684278 | -0.325406 | -0.676476 | 0.438680 | 0 |
1 | 3 | 0.997273 | -0.321107 | 0.112882 | -0.215089 | 0.183626 | -0.325406 | 0.417193 | 0.636132 | 2 |
2 | 7 | 2.568161 | -0.583534 | 2.413358 | -0.124285 | -0.684278 | -0.325406 | 5.044256 | -0.005927 | 2 |
3 | 14 | 2.165449 | -0.583534 | -0.845650 | -0.124285 | 0.698944 | -0.325406 | 1.707162 | -0.480852 | 2 |
4 | 21 | 0.203260 | -0.583534 | 0.783854 | -0.896118 | 1.160018 | -0.325406 | 0.753707 | -0.572470 | 0 |
... | ... | ... | ... | ... | ... | ... | ... | ... | ... | ... |
4480 | 56472 | -0.273010 | 0.372450 | 0.318282 | -0.124285 | -0.157336 | 0.063454 | -0.063540 | 0.564427 | 0 |
4481 | 56488 | 2.528641 | -0.583534 | -0.845650 | -0.896118 | -0.684278 | -0.325406 | 1.230435 | 1.578633 | 2 |
4482 | 56498 | 0.896734 | 1.647094 | 0.783854 | 1.419380 | 1.160018 | 1.035602 | 0.515343 | -0.171906 | 2 |
4483 | 56505 | -0.287742 | 3.877723 | -0.845650 | -0.124285 | -0.684278 | -0.325406 | -0.676476 | -0.744070 | 0 |
4484 | 56507 | 0.064449 | 1.200969 | 1.109755 | 0.801914 | 0.053441 | 0.763400 | 0.753707 | 1.506299 | 2 |
4485 rows × 10 columns
Step3. Select clustering method and number of clusters¶
list(range(2, 11))
[2, 3, 4, 5, 6, 7, 8, 9, 10]
df_cluster_scorer = pd.DataFrame()
df_cluster_scorer['n_clusters'] = list(range(2, 21))
def score(n_clusters, df, features):
km = KMeans(n_clusters=n_clusters, max_iter=300, random_state=None)
X = df[features]
labels = km.fit_predict(X)
SSE = km.inertia_
Silhouette = metrics.silhouette_score(X, labels)
CHS = metrics.calinski_harabasz_score(X, labels)
DBS = metrics.davies_bouldin_score(X, labels)
return {'SSE':SSE, 'Silhouette': Silhouette, 'Calinski_Harabasz': CHS, 'Davies_Bouldin':DBS, 'model':km}
score(3,city_groups_std, num_list)
{'SSE': 27710.665681039078,
'Silhouette': 0.2627460302851151,
'Calinski_Harabasz': 660.6663081740991,
'Davies_Bouldin': 1.7603028939928969,
'model': KMeans(n_clusters=3)}
df_cluster_scorer['SSE'],df_cluster_scorer['Silhouette'],\
df_cluster_scorer['Calinski_Harabasz'], df_cluster_scorer['Davies_Bouldin'],\
df_cluster_scorer['model'] = zip(*df_cluster_scorer['n_clusters'].map(lambda row: score(row, city_groups_std, num_list).values()))
df_cluster_scorer
n_clusters | SSE | Silhouette | Calinski_Harabasz | Davies_Bouldin | model | |
---|---|---|---|---|---|---|
0 | 2 | 31356.632019 | 0.251428 | 646.700214 | 2.102368 | KMeans(n_clusters=2) |
1 | 3 | 27710.643740 | 0.262784 | 660.670386 | 1.760638 | KMeans(n_clusters=3) |
2 | 4 | 25274.767410 | 0.247363 | 626.741372 | 1.594434 | KMeans(n_clusters=4) |
3 | 5 | 23028.000458 | 0.265498 | 625.076225 | 1.460516 | KMeans(n_clusters=5) |
4 | 6 | 21442.540001 | 0.273858 | 603.152500 | 1.362168 | KMeans(n_clusters=6) |
5 | 7 | 20084.386296 | 0.154472 | 586.966244 | 1.438337 | KMeans(n_clusters=7) |
6 | 8 | 18904.542702 | 0.163691 | 574.308025 | 1.394910 | KMeans() |
7 | 9 | 17749.043623 | 0.168539 | 571.539792 | 1.369433 | KMeans(n_clusters=9) |
8 | 10 | 16963.355795 | 0.174827 | 554.476130 | 1.326198 | KMeans(n_clusters=10) |
9 | 11 | 16430.618280 | 0.174942 | 529.602262 | 1.401950 | KMeans(n_clusters=11) |
10 | 12 | 15692.925235 | 0.183482 | 523.091274 | 1.362996 | KMeans(n_clusters=12) |
11 | 13 | 15162.305849 | 0.167418 | 509.209753 | 1.403024 | KMeans(n_clusters=13) |
12 | 14 | 14726.863496 | 0.127382 | 494.000916 | 1.468960 | KMeans(n_clusters=14) |
13 | 15 | 14244.740729 | 0.164447 | 484.939934 | 1.421487 | KMeans(n_clusters=15) |
14 | 16 | 13940.977401 | 0.141971 | 468.860714 | 1.476356 | KMeans(n_clusters=16) |
15 | 17 | 13637.438010 | 0.172236 | 455.455632 | 1.422667 | KMeans(n_clusters=17) |
16 | 18 | 13259.476331 | 0.156201 | 448.276811 | 1.398571 | KMeans(n_clusters=18) |
17 | 19 | 13042.270028 | 0.145194 | 434.458776 | 1.408099 | KMeans(n_clusters=19) |
18 | 20 | 12738.974719 | 0.172705 | 426.890002 | 1.372609 | KMeans(n_clusters=20) |
df_cluster_scorer.plot.line(subplots=True,x ='n_clusters', figsize=(12,12))
array([<AxesSubplot:xlabel='n_clusters'>,
<AxesSubplot:xlabel='n_clusters'>,
<AxesSubplot:xlabel='n_clusters'>,
<AxesSubplot:xlabel='n_clusters'>], dtype=object)
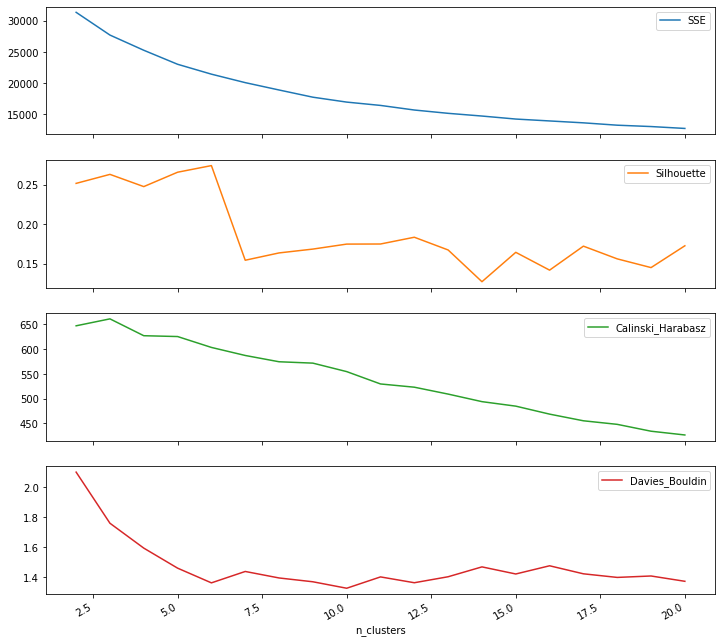
df_cluster_scorer.plot.line(y='SSE',x ='n_clusters',logy=True, figsize=(12,3))
<AxesSubplot:xlabel='n_clusters'>
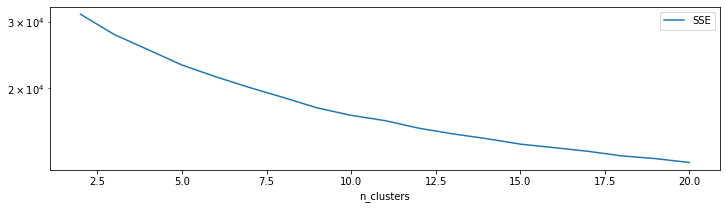
Visualize the cluster¶
pca = PCA(n_components=2, whiten=True)
pca.fit(city_groups_std[num_list])
PCA(n_components=2, whiten=True)
pca.fit_transform(city_groups_std[num_list])
array([[ 0.09362892, -0.52372539],
[ 0.84601865, -0.14918922],
[ 3.82881756, -0.1731645 ],
...,
[ 0.46338984, 1.53211431],
[-0.97116177, -0.50927138],
[ 1.16965175, 1.06541916]])
city_groups_std['x'], city_groups_std['y'] = zip(*(pca.fit_transform(city_groups_std[num_list])))
plt.scatter(city_groups_std['x'], city_groups_std['y'], c=city_groups_std['cluster'])
plt.show()
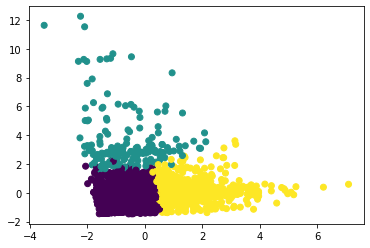
df_cluster_scorer[df_cluster_scorer['n_clusters']==2]['model'].values[0]
KMeans(n_clusters=2)
@interact
def show_clusters(n=(2,20)):
model = df_cluster_scorer[df_cluster_scorer['n_clusters']==n]['model'].values[0]
labels = model.predict(city_groups_std[num_list])
plt.scatter(city_groups_std['x'], city_groups_std['y'], c=labels)
plt.title(f"Cluster {n}")
plt.show()
Step4. Profile the cluster¶
city_groups.merge(city_groups_std[['user_location_city', 'cluster']]).groupby('cluster').mean()
user_location_city | orig_destination_distance | is_mobile | is_package | srch_adults_cnt | srch_children_cnt | srch_rm_cnt | duration | days_in_advance | |
---|---|---|---|---|---|---|---|---|---|
cluster | |||||||||
0 | 28059.919851 | 1390.514992 | 0.137752 | 0.184819 | 1.994703 | 0.377777 | 1.069875 | 2.794248 | 42.493643 |
1 | 31017.981928 | 1525.042593 | 0.115663 | 0.196687 | 4.084940 | 0.567671 | 2.412249 | 3.069378 | 55.478239 |
2 | 28893.937273 | 3189.285509 | 0.112741 | 0.487444 | 2.029138 | 0.321586 | 1.069821 | 5.300009 | 101.977415 |
Step 5: assess the statistical robustness¶
A statistically robust segmentation return similar results using different clustering methodologies